The Faculty of Engineering Represented at the ICLR 2021
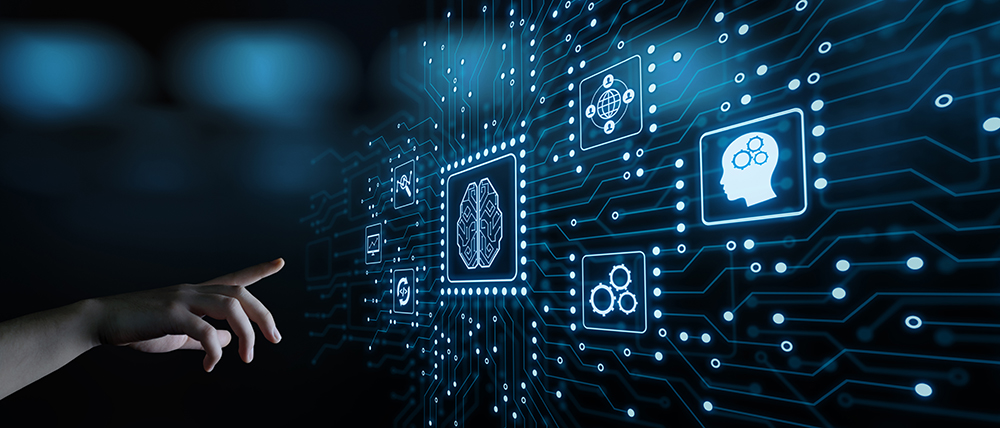
The ICLR 2021 (International Conference on Learning Representations) is one of three leading conferences in the world of computational learning. To be held this May, the conference will feature two projects from the Faculty of Engineering, conducted by students co-guided by the Faculty’s own Dr. Ethan Fetaya, and Prof. Gal Chechik of the Leslie and Susan Gonda (Goldschmied) Multidisciplinary Brain Research Center. This is an important achievement, considering that only 860 of the 3,000 applications were accepted.
The first paper, Auxiliary Learning by Implicit Differentiation, explores learning via auxiliary tasks. “The paper examines how we can use auxiliary tasks to reach optimal results when the main tasks have nearly no tags,” explains Dr. Fetaya. “Let’s take a task of precise identification of bird species. There are hundreds of species, and only an expert can tell the exact difference between, say, a red-necked stint and a long-toed stint. But we can use simpler auxiliary tasks that can help with the identification: the shape of the beak, or color of the wings. These are tasks that any reasonable person can execute, and are much easier to identify. The paper shows that even without many tags on the main task, we were able to achieve a significant improvement in performance thanks to the auxiliary tasks.” The research was headed by doctoral students Aviv Navon and Idan Ahituv, Dr. Fetaya, Prof. Chechik, and Dr. Haggai Maron of the Nvidia research group.
The second paper, Learning the Pareto Front with Hypernetworks, was written by Dr. Fetaya, Prof. Chechik, and doctoral students Aviv Navon and Aviv Shamsian. It focuses on minimizing mistakes while multitasking. “When I’m trying to perform several tasks at once, the goal is to execute all of them optimally and avoid mistakes as much as possible. It’s not always an easy task: for instance, I could be trying to screen candidates to a certain position according to education, knowledge, and experience, but I also want to avoid gender or nationality discrimination,” says Fetaya. “Usually, when it comes to a combination of complex tasks, there’s no single optimal solution – there’s a series of tradeoffs that the algorithm might suggest: achieve a very good result in one task, a mediocre result in the second and third tasks, and a terrible result in the fourth. But you don’t usually know beforehand which result you want: you want to know the alternatives and be able to choose from them. Until now, the only way to do that was to run a lot of models and look at the results – expensive and takes a long time. Our paper suggests using hyper networks, a method that tries to learn all possible outcomes simultaneously. That way, we can train a single joint model to retrieve all the alternatives, then choose the one that best suits your needs.”
Last Updated Date : 14/02/2021