Mission: Promote Science
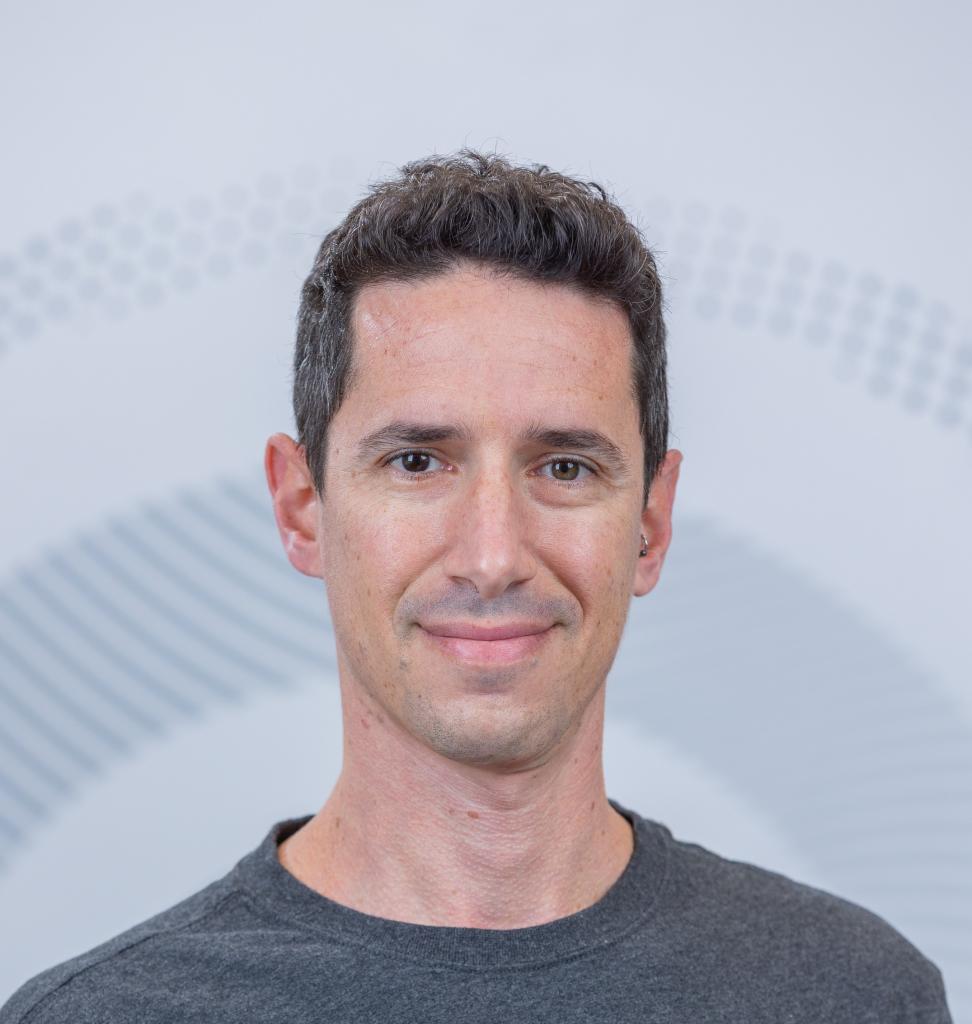
Meet Dr. Ofir Lindenbaum, who specializes in Machine Learning and develops algorithms that can improve scientific research in almost any field—from discovering new drugs for cancer, to predicting floods
The past years have seen a significant improvement in everything related to cancer treatment. Scientific breakthroughs have led to the discovery of innovative treatments such as biological protocols, based on changing genes understood by biologists to affect the immune system’s response to tumors. But there is always room for (significant) improvement. “Machine Learning will help us find dozens, if not hundreds, of these types of genes which are currently hidden,” says Dr. Ofir Lindenbaum, “and detecting them could lead to the development of new drugs that are personalized and much more effective than the current offering—biologically and globally speaking.”
Lindenbaum (38) specializes in Machine Learning and joined the Faculty of Engineering in October of 2021. He earned his BSc in Physics and Electrical Engineering from the Technion, and his MSc and PhD in Electrical Engineering from Tel Aviv University. “Early in my research I studied signal processing, and that’s how I found myself in Machine Learning. All that time, I was intrigued by a single question above all others: How to extract the specific scientific information we need from the sampled data at our disposal,” he shares. “For my PhD thesis, I studied multifaceted data integration—data from computers and different gauging devices such as audio and images from X-rays and MRIs. My goal has always been to learn how to use Machine Learning to promote all forms of scientific research, from medicine and biology to geophysics.”
He did his post-doctoral research on applied mathematics at Yale. “For my post-doctoral studies, I mainly focused on data in the field of biology and medicine. At Yale I worked mainly with researchers from the faculties of medicine and biology; they would come up with a scientific question and we would try to solve it by collecting data using computers that sample single cells, or imaging tests of patients, and figure out what factors affect the issue,” he explains. “My role was to develop tools that use Machine Learning for automation, to identify patterns and variables that affect medical problems. I spent 3.5 years at Yale, but my last year was done here, in light of Covid. The pandemic enabled analytical people such as myself to work remotely.”
He joined the Faculty of Engineering in October of 2021 and found his place in the data engineering track. He then formed his group, Machine Learning for Scientific Discovery, which develops Machine Learning tools. The group develops Machine Learning tools to help automatically research scientific questions. “My group is very diverse,” he says. “We all develop tools for solving practical scientific problems, but in different fields. In my lab, we study issues pertaining to psychology, biology, and neuroscience. One collaboration with Dr. Vadim Axlerod of the neuroscience research center attempts to predict the consciousness of people in a vegetative state, using fMRI. Another collaboration, with Prof. Sharon Granot of the Faculty of Engineering, focuses on speech signals. A third collaboration, this time with Dr. Jonathan Granot of the Geography Department, is all about predicting urban flooding.”
Dr. Lindenbaum is also developing a new course for master’s students, on unsupervised learning. “Unsupervised learning is a type of learning that does not require human tagging. In Machine Learning, a significant part of research is based on tagged examples—the kind that requires human tagging to enable us to train the Machine Learning models, such as images that we know to show the face of a specific person or audio recordings where somebody says a specific world,” he explains. “Conversely, models that do not require tagging seek patterns within databases—patterns that even humans may not be able to detect. For example, searching a table of hundreds of genes for certain genes that affect a specific medical problem. Humans struggle to find patterns in data of this kind, especially if the chart is not properly organized. Another example of this combo can be found in my joint study with Dr. Jonathan Ganot, on predicting floods. Our goal is to be able to provide citizens of cities in the Dan metropolitan area with real-time alerts as a means of preventing incidents of flooded vehicles or inhabited basements. We measure data from the meteorological service and match it with citizen reports to produce on-time predictions. We’re at the model building stage, and it’s based on tagged examples—but when the time comes, we would like to use it on untagged examples, to predict floods before they are reported.”
Dr. Lindenbaum is currently recruiting MSc and PhD students to work on various Machine Learning projects. “In my group, research is divided into algorithm development—which requires mathematical understanding and analytic skills—and working with real data, which requires comprehension and intuition regarding the type of problem and patterns—a task best suited for students with a biological or medical background. Either way, I want to work with people who are curious, and who enjoy research. My dream is to be able to develop tools that could fit every scientific field: to have biologists, physicians, and geophysicists, for example, use our tools and to have our tools help them make new discoveries that cannot be made using the tools currently at our disposal. I see myself doing this in the future, as well, to change the way scientists think. I want to improve science, give it a nudge forward and help make new discoveries.”
More on Dr. Lindenbaum’s work can be found here
Last Updated Date : 23/05/2022