Prof. Zeev Zalevsky’s In-Fiber Optical Neural Network
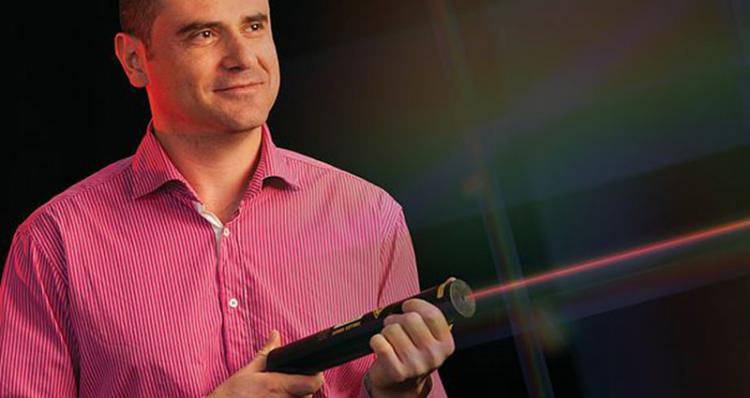
Artificial neural networks seek to mimic – in silico – what the biological brain does naturally: real-time parallel processing of massive data sets. Now, the Engineering Faculty’s Prof. Zeev Zalevsky, together with post doctoral researcher Dr. Eyal Cohen and Zalevsky’s colleague from Hebrew University Dr. Mickey London, has presented the first-ever conceptual design for an in-fiber optical neural network – a portable, photonic processor in which light-based signals are shared within a “feed forward” neural network computational structure. Not only does this patent-pending system demonstrate high-speed parallel processing with low power consumption, it also achieves something that we take for granted when it happens between our ears: by taking in and processing external data, this optical neural network can learn.
“Optical neural networks have many potential advantages, such as the ability to transfer data from single points to many targets, and to do so without the massive heat generation of traditional electronics,” says Zalevsky, who serves as head of the Electro-Optics study program at the Faculty of Engineering and also directs the Nano Photonics Center at BIU’s Institute of Nanotechnology and Advanced Materials (BINA). “However, optical networks’ large size and lack of scalability have so far prevented progress. Taking advantage of recent advances in fiber fabrication, we’ve overcome these difficulties.”
Zalevsky’s is the first-ever system to achieve learning based on external inputs – all in an optical “computer” thinner than a human hair.
A photonic system in which externally-pumped energy sources direct optical signals toward a three-layer array of silica cores – some of which are doped with an all-optical amplification element called erbium – Zalevsky’s model achieves more than just the controlled transfer of light. Simulations suggest that the network can process light-based information, differentiating between, and classifying, various input patterns.
“The in-fiber network can impose an artificial classification on the optical signals it receives,” Zalevsky explains. “This is good news – it means that such a system could potentially be used as a basic building block for the large-scale optical networks of the future.”
Compact structure, high speed and low power consumption makes in-fiber optical processing an ideal candidate for heavy computational challenges like bitcoin mining – a network-based process through which distributed resources are put to work solving mathematical problems, in exchange for payment in digital currency. And according to Zalevsky, the true power of the system has yet to be revealed.
“Ours is a simple model, based on relatively small number of embedded silica cores,” he says. “Improvements in fabrication techniques could lead to much more complex systems, in which each a fiber has tens of thousands of optical ‘input’ cores. Eventually, our goal is to design an all-optical network within fibers, where a built-in learning algorithm leads to robust computational results.”
Last Updated Date : 06/09/2017