Speaker Localization in Reverberant Rooms
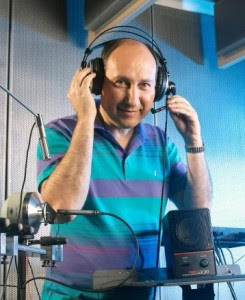
Professor Sharon Gannot and his team address this classic problem in the field of signal processing, by methods of manifold learning
Last February, Prof. Sharon Gannot gave a keynote lecture at the LVA-ICA conference held in France. Gannot, 52, who specializes in speech signal processing, referred in his lecture to the problem of speaker localization in reverberant rooms. "Usually, when a person speaks in a room or a closed space, we hear it both directly and as a returning echo from objects in the room. This phenomenon, called reverberation, affects the ability to recognize the location of the speaker, because the voice signal actually comes from many places at the same time. This is a classic problem in signal processing, and it is relevant when you want to pinpoint the exact location of speakers - for example, when you want to automatically turn a camera on to a lecturer speaking on stage, or to a speaker in a conference room."
"Most of the methods that tried to deal with this problem tried to ignore or erase these reflections, and focus on direction of arrival only. We tried to contend with the problem in a different way, and essentially use the pattern of reflections for a more precise location. Our method maintains that the pattern of reflections is characteristic of locations in the room, i.e., each location in the room has its own 'fingerprint'. Our goal is to identify the location from that pattern. Apparently, it sounds simple; just scan all possible fingerprints, and find the most appropriate one. However, that could not work because the reflection pattern is very complicated and complex. Furthermore, if the acoustic conditions change slightly, for example, if a piece of furniture is moved from its place or a window is opened, the pattern of reflections may change in a way that will render it unidentifiable. Therefore, we are searching for a method that can measure the 'significant' distances between these patterns. We do this using a method termed dimensionality reduction: We look for a method that will extract the dominant parameters, or the "essence" of these patterns, and then link between this essence and the location."
"For this to happen, we are exploring methods to organize these patterns, that is, to make order in large dimension patterns. To this end, we took advantage of a method called 'manifold learning'. This method describes the pattern space as a 'manifold', and can organize the patterns on it in such a way that the measurement of the distance between them is significant. This can be visually compared to a 'flying carpet': when the carpet is hovering over a room, it is three-dimensional. It receives waves and curves, but in practice it is two-dimensional. So when we measure the distance between points in a straight line, we obtain a meaningless distance. In order to measure a meaningful distance, we'll have to flatten the carpet on the floor and measure distances with a ruler. A similar principle can be used to measure distances on manifolds. If we want to measure a significant distance between points on the manifold, we must respect its curves."
"Now, the question is - how do we discover the shape of the manifold? As mentioned, in order to locate a speaker, we have to take familiar patterns of reflections and compare them with a pattern of reflections that characterize a position we are interested in locating. We cannot, of course, measure all the points in the room; that would be exhausting work. Therefore, we use a combination of supervised learning and unsupervised learning. In the first stage, we learn a small number of points in an accurate manner. During learning, we refer to the matches between a location and a reflection pattern. A small number of training points (meaning points measured accurately before applying the algorithm) is not enough to identify all the curves on the manifold, but they serve as anchors. In the second, unsupervised phase, we add more and more points from the same room, and thus we receive multiple reflection patterns that help us infer the structure of the manifold and its curves. After a certain period of time in which the room has been studied and information has been gathered about the location of multiple points, it will be easy to locate each new point, because, on the one hand, the curves on the sheet have been inferred, and on the other hand, the anchor points, which have been inferred in a supervised manner, have anchored it in space. Thus, when a new source from an unknown location in the room has been measured, we can identify where it is located within this space, by comparing it with all the points that have been accumulated up until now."
The question arises as to whether it is possible to assume, in realistic problems, the existence of training information as required. Gannot claims that collecting the necessary information is absolutely possible, and he relies on the fact that much of our professional life takes place in set places, such as our office or classroom. However, of course - in a new room, we will always need the initial measurement, which gives us an these anchor points.
The method, which was developed in the framework of Bracha Laufer-Goldstein's doctoral dissertation, under the guidance of Prof. Gannot and Prof. Ronen Talmon of the Technion, demonstrates improved performance as compared to classic approaches for localization in reverberant rooms, and can also be expanded for tracking a speaker moving in a room.
Last Updated Date : 06/09/2017